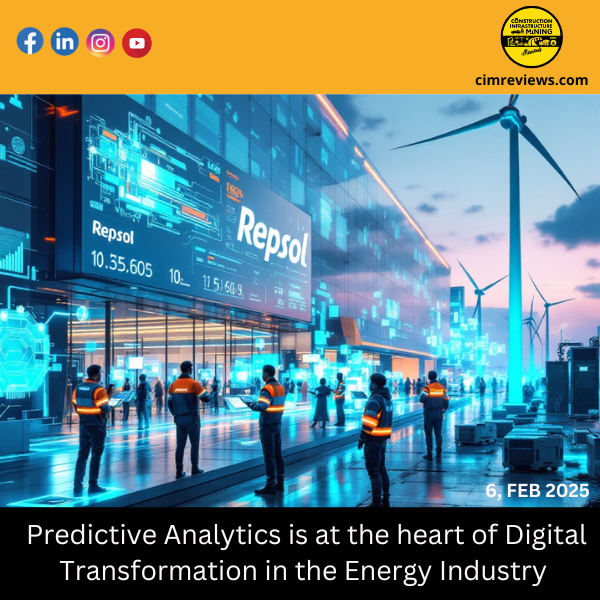
Digital Data Management expert Senthilkumar Pandi highlights the importance of Predictive Analytics in the Energy Industry for preventing costly downtimes and ensuring the maintenance of multi-billion dollar assets. This technology helps detect faults and provide early warnings in critical rotary equipment like compressors, pumps, motors, turbines, fans, blowers, and gearboxes.
Predictive Analytics is a crucial tool for organizations to prevent equipment failures and reduce unnecessary maintenance. To implement the right predictive analytics solution, organizations must consider several factors, including compatibility with various sources, modern and user-friendly interfaces, compatibility with data encryption/data protection policies, and the ability to generate notifications and alerts based on data-driven techniques like statistical models and AI and Machine Learning.
To ensure the reliability of predictive analytics, organizations should evaluate standard libraries for various types of equipment and the OOTB model available for various use cases. Standard industry software such as AVEVA Predictive Analytics, Hexagon EAM, and Baker Hughes Advanced ESP Predictive Failure Analytics are widely adopted by the Energy Industry to deploy Predictive Analytics to protect critical assets.
Hardware and software selection is also essential for successful implementation of predictive analytics. A robust hardware and supporting OS and other applications are necessary for fetching, integrating, and synthesizing data for analysis. Before implementing the system, a thorough investigation on the usability of existing data available historically should be conducted.
Alarm/Alerts will start generating when there is a deviation from the predictive model, allowing operators to identify the root cause of the deviation well in advance. Traditional alarms set based on threshold values may not be sufficient to prevent costly damage to the asset.
Deployment methodology and KPIs of the system are crucial for ensuring data security. An idle predictive analytics solution should be deployed on the premises to avoid incidents related to data security. Security testing should be conducted using VAPT tool or through CERT, and system performance should be linked to various KPIs to realize the benefits.
Predictive Analytics is at the Heart of Digital Transformation in the Energy Industry
The energy industry is undergoing a digital revolution, with predictive analytics playing a central role in optimizing operations, improving efficiency, and reducing costs. By leveraging vast amounts of data and advanced algorithms, predictive analytics enables energy companies to anticipate demand, detect potential failures, and enhance decision-making.
One of the most significant applications is in grid management and energy forecasting. By analyzing historical consumption patterns, weather data, and real-time sensor inputs, energy providers can predict demand fluctuations with high accuracy. This allows for better load balancing, reducing energy waste and improving grid stability.
Predictive maintenance is another game-changer. Energy companies rely on extensive infrastructure, including power plants, wind turbines, and transmission lines. Predictive analytics, powered by AI and IoT sensors, helps identify early signs of equipment failure, allowing for proactive maintenance. This reduces downtime, extends asset lifespan, and prevents costly outages.
In the renewable energy sector, predictive analytics enhances efficiency by optimizing wind and solar energy production. Weather forecasting models help predict sunlight and wind speeds, ensuring maximum energy capture and grid integration. This is crucial for increasing the reliability of renewable energy sources and reducing dependency on fossil fuels.
Additionally, fraud detection and cybersecurity are benefiting from predictive analytics. By continuously analyzing transactional and network data, energy companies can detect anomalies and prevent cyber threats, ensuring the security of critical infrastructure.
As the energy industry continues its digital transformation, predictive analytics will remain at the core of innovation. By harnessing the power of big data AI and energy companies can build a smarter, more sustainable, and resilient future, meeting growing energy demands while minimizing environmental impact.